As the search for more elusive particles requires more precision and creative approaches, Fermilab scientist Kevin Pedro is using the power of artificial intelligence to look for evidence of new physics in unexpected places.
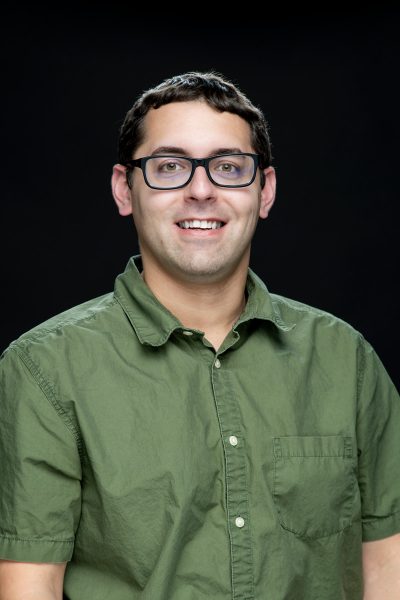
Kevin Pedro
Pedro received this year’s Universities Research Association Honorary Early Career Award, which recognizes outstanding work in cosmology, particle astrophysics and high-energy physics by young researchers at or in collaboration with Fermilab. His research includes looking for signs of dark matter and developing new machine learning techniques.
“Kevin Pedro’s program is built on a unique ability to translate his depth of technical and physics knowledge into prolific and broadly impactful research,” said Cecilia Gerber, distinguished professor in the Department of Physics at the University of Illinois Chicago and current chair of the URA Early Career Award committee. “With a core belief in supporting fellow physicists, Kevin is very invested in developing and supporting valuable and practical tools used by researchers in other subfields. Kevin is also a committed mentor and an active advocate in public outreach. He is very deserving of the 2024 URA award recognition.”
URA president and CEO John Mester added, “Dr. Pedro’s work is pushing the boundaries of human knowledge and technological capability. Our understanding of the cosmos will potentially reveal new particles and interactions beyond the Standard Model, which will drive the development of novel detectors with unprecedented sensitivity and advanced computational methods, and foster interdisciplinary approaches needed to address these complex scientific questions.”
Dark matter is an invisible form of matter that physicists have evidence for but have never detected directly. Astronomers first inferred its existence when observations of the gravitational behavior of galaxies implied that they contained more mass than could be seen. Many searches for dark matter look for hypothetical fundamental particles, like WIMPs or axions, but none have been discovered yet. Instead, Pedro’s research looks for signs of composite particles, like dark matter versions of hadrons such as protons and neutrons, in the Large Hadron Collider’s CMS experiment.
“These models tend to look very different from the standard picture of dark matter being a single particle,” Pedro said.
When composite particles like protons collide in a particle accelerator, the individual quarks making up the protons and the gluons that hold them together are knocked out of place. Since single quarks and gluons cannot exist on their own, they produce fountains of more hadrons in a phenomenon known as a jet.
Similarly, collisions that produce the hypothetical dark versions of quarks would lead to jets of dark matter hadrons, which would be observed as semivisible jets if some of the dark matter particles are unstable enough to decay into known visible particles.
“Depending on how they decay and when they decay, you get different signatures, which are unlike anything that you’d normally see in Standard Model events,” Pedro said.
These semivisible jets would look like a typical jet, but with missing parts. However, it would be hard to tell the difference between a genuine semivisible jet and a normal jet observed with a malfunctioning detector or reconstruction algorithm.
“If it were easy to pick out these things in the data, we might have already found it, but we haven’t found it,” Pedro said.
These events where parts of the jet seem to be missing are usually just discarded and dismissed as malfunctions or detector noise. Pedro’s work looks for better ways to reject background noise to pick out the true background noise from potential signals of semivisible jets.
“We’re searching where people consider the background is,” he said. “You have to reject these dead areas of the detector more precisely.”
Additionally, if dark matter comprises composite particles — also called dark hadrons — rather than fundamental particles, understanding the parameters at play becomes more complicated. A single fundamental particle can be characterized by its mass and its interactions with other particles; however, dark hadrons would require multiple fundamental particles, more decay options and a new force to hold these composite subatomic particles together.
“There are a ton of parameters, and we don’t know what any of those parameters should be,” Pedro said. “It’s a massive space to explore, too big to completely explore exhaustively with the millions of different models you’d have to try.”
To solve these problems, Pedro is exploring the use of artificial intelligence and machine learning. One technique is known as anomaly detection. This involves training the machine learning model to recognize events consistent with the Standard Model of particle physics. Then, the algorithm attempts to reconstruct the new events it sees, in order to identify the events that couldn’t be properly reconstructed.
Although this method isn’t as sensitive to specific models of new physics, it casts a wider net than training an algorithm to look for evidence for a particular model.
“You don’t have to specify 12 or 15 model parameters,” Pedro said. “You just need a very broad idea of what you want, and then it can reject background and identify the signal for any model that fits.”
However, running these machine learning algorithms can take up valuable time, so Pedro also researches ways to speed them up. This becomes important when sorting events at the Large Hadron Collider, where the trigger system only has microseconds to decide whether or not to save an event’s data.
Pedro’s solution is to delegate the algorithm’s workload to a separate, more powerful machine, a process called inference as a service.
“You can use all the resources throughout the CMS collaboration and get an answer much faster,” he said. “This abstraction lets us scale up a lot of this work beyond what we could do with limited resources.”
Another situation where computing bottlenecks arise is simulating how events look in the detectors. Here, generative machine learning can help, such as image-generating algorithms that work similarly to the currently popular text-generation programs or image-diffusion models.
Pedro’s research takes this idea one step further by training the algorithm to learn what the data contains. The algorithm learns the unique geometry of the detector, rearranges the geometry in a way that makes sense to the algorithm, determines the properties of the data and then generates a simulated event.
“We found that it’s almost indistinguishable from the real simulation that uses all the physics,” Pedro said. “We’re trying to get rid of the ‘almost’ now.”
Such methods will be crucial after the LHC’s next upgrade. The High-Luminosity LHC will gather higher volumes of complicated data that will require time to process.
“We will have bigger problems; we want to do more precise physics, so we’re going to be employing artificial intelligence more,” Pedro said.
The LHC isn’t the only experiment that could benefit from these machine learning approaches. When the prototype detector for Fermilab’s DUNE experiment was tested at CERN, machine learning as a service helped to analyze the heaps of data it gathered, cutting the analysis time from months to weeks, Pedro said.
“It has broad applications across high-energy physics and beyond to use resources flexibly,” he said.
And this work could be used to study images in astrophysics, Pedro said — right back where this hunt for dark matter began.
“There’s stuff we don’t understand. We know it’s out there, maybe it’s down here, too,” he said. “We’re trying to look everywhere we can look.”
Fermi National Accelerator Laboratory is supported by the Office of Science of the U.S. Department of Energy. The Office of Science is the single largest supporter of basic research in the physical sciences in the United States and is working to address some of the most pressing challenges of our time. For more information, please visit science.energy.gov.