To solve mysteries of the universe, scientists use powerful particle accelerators and detectors. These enormous machines generate millions of particle collisions and petabytes of data every second. The sheer amount of data outpaces the rate at which the detector can transfer it — a growing problem with even more advanced experiments on the horizon.
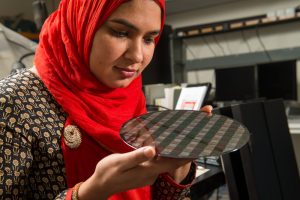
Farah Fahim is one of the first engineers to win the DOE Early Career Research Award in high-energy physics. Photo: Fermilab
The U.S. Department of Energy awarded Fermilab engineer Farah Fahim, Ph.D., an Early Career Research Award to work on a solution. She’ll apply the $2.5 million grant to her work, which uses neural networks and machine learning to create efficient hardware for data processing at source. Converting the raw data locally into higher-level, lower-volume information overcomes the data transfer bottleneck. In addition, it enables real-time data-processing, which will accelerate discoveries.
Fahim’s efforts center on the Large Hadron Collider, the 27-kilometer particle accelerator at CERN. There, protons smash together and produce other particles that whiz through a detector. One component, the pixel detector, is the size of a shoebox. It’s layered with 65 million pixels that pick up the particles’ movements. Knowing which pixels a particle touched tells researchers about its trajectory.
But with so many pixels and particle paths to map, the issue of creating too much data too quickly to process arises. Fahim wants to see whether using neural networks in small areas within the pixel detector can help, a technique called “artificial intelligence on-chip.”
Neural networks use a series of processing “nodes,” each transmitting a tiny piece of information that builds into an informative picture. Machine learning could teach a neural network to identify shapes or patterns and help them recognize the particles as they pass through the pixel detector. The ML algorithm would allow it to send information about interesting particle paths to researchers without having to send out raw data from each pixel, overcoming the communication challenge.
“Instead of sending this voluminous amount of data even to the edge of the chip and then processing it, can we just do this right in the beginning, within the pixel where the data is generated, and get some useful information?” Fahim said.
Neural networks are often huge powerful circuits made up of hundreds of nodes. But Fahim will use several smaller, more energy-efficient networks that specialize in data classification to recognize patterns in the pixel detector. She will try different techniques for compact on-chip AI, including using nanoscale materials to add electrical components called “memristors.” Scaling down neural networks and AI technology to fit directly on a chip means adding intelligent data processing into small areas, right in the detector.
“The long-term objective is real-time particle track reconstruction,” said Fahim, though she knows that goal might be beyond the scope of the award, which funds research over five years. “We will contribute one drop of knowledge about how to locally analyze this information and how to transform the data.”
In addition to experiments at the LHC, Fahim’s research could have applications in experiments and facilities at several U.S. national labs. They include the international Deep Underground Neutrino Experiment hosted by Fermilab; the Electron Ion Collider hosted by Brookhaven National Laboratory; and at light sources, such as LCLS-II at SLAC National Accelerator Laboratory. For Fahim, the award is an acknowledgment that her work creating new, efficient data-processing hardware is becoming increasingly valuable. She is one of the first engineers to win the award in high-energy physics.
Fahim has a doctorate in electrical engineering and has worked at Fermilab for 12 years, developing pixel detectors for a variety of applications. She became the deputy head of Fermilab’s quantum science program in 2019. She is also the head of the Application Specific Integrated Circuit, or ASIC, research and development department, which focuses on building circuits for potential application in detectors, quantum, AI and 5G/6G communication.
“I am impressed by her entrepreneurial and innovative approach, and the fact that she’s not afraid to try new things,” said Panagiotis Spentzouris, head of Fermilab’s quantum science program. “She puts in a lot of effort, and the combination of hard work, technical expertise, leadership and team-building skills, and innovative ideas is what will solve this multifaceted challenge.”
Fermilab is supported by the Office of Science of the U.S. Department of Energy. The Office of Science is the single largest supporter of basic research in the physical sciences in the United States and is working to address some of the most pressing challenges of our time. For more information, please visit science.energy.gov.