Jennifer Ngadiuba has been fascinated by the universe since a young age. “I had binoculars to watch the stars,” said Ngadiuba, a Wilson Fellow at the Department of Energy’s Fermi National Accelerator Laboratory. But it was fundamental physics which grabbed her heart. “In high school the teacher gave us a book by Stephen Hawking, and I decided that’s what I wanted to do.”
Recently, Ngadiuba’s contributions to particle physics were recognized by the International Union of Pure and Applied Physics, who awarded Ngadiuba the 2024 Early Career Scientist Prize in Particle Physics.
“This award is one of the most prestigious worldwide,” said Anadi Canepa, the head of the Fermilab CMS group and the incoming deputy spokesperson of the CMS experiment. “It’s a testament to Jennifer’s vision, drive and her interdisciplinary approach to physics. She is a trail blazer and an outstanding physicist, and we are extremely proud of her achievement.”
Ngadiuba received the award on July 24, 2024, during a ceremony at the International Conference on High Energy Physics in Prague. In the announcement, IUPAP cited Ngadiuba’s contributions to ultra-fast machine learning techniques and their application to anomaly detection.
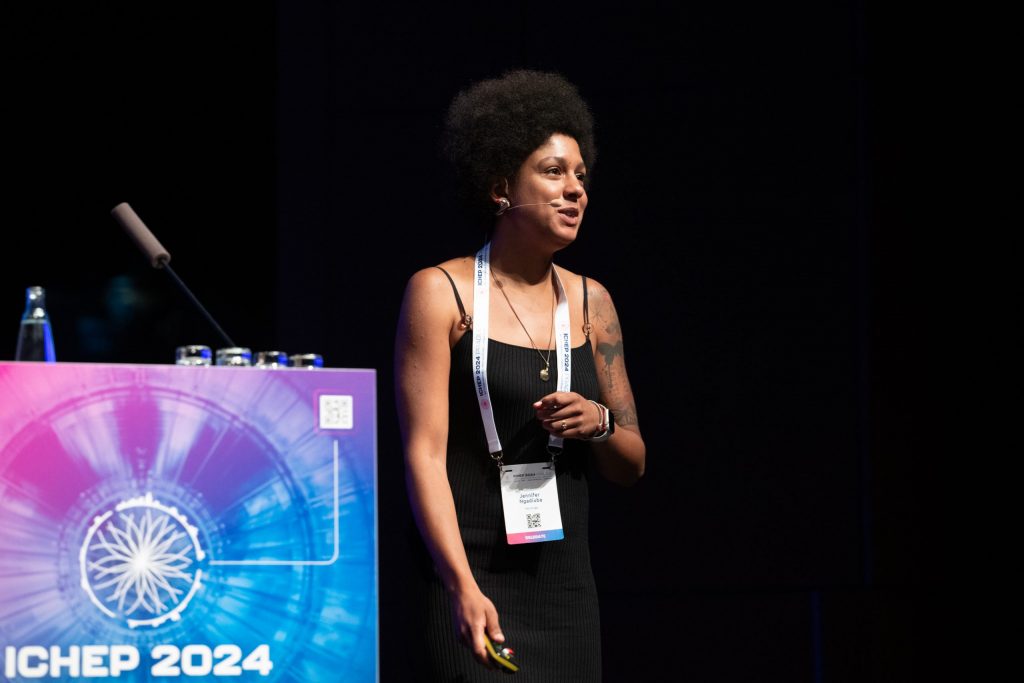
Jennifer Ngadiuba presents during the International Conference on High Energy Physics in Prague, where she was awarded the Early Career Scientist Prize in Particle Physics. Photo credit: Rene Volfik
“Jennifer is using AI to reshape collider physics,” said Maurizio Pierini, the CMS physics coordinator. “I expect that in ten years from now, CMS and ATLAS will do things very differently from the way we do today and, looking back, we will track much of this evolution — which is actually an AI revolution — back to her work.”
During college, Ngadiuba developed a passion for experimental tools. “When I was studying physics, it was a lot of math, a lot of theory, and a lot of quantum mechanics,” she said. “I thought, I need to understand how we actually see all this. What is the instrument that allows us to make a theory not a theory?”
This curiosity led Ngadiuba to Fermilab, where as a master’s student she worked on tracking detectors R&D for the future upgrade of the Compact Muon Solenoid experiment, one of the two general purpose detectors at the Large Hadron Collider. But it was during a fellowship at CERN when she was captivated by machine learning.
“Things were changing in society,” she said. “There was this explosion of data in both science and industry.”
Machine learning has been used in physics analyses since the 1980s, but Ngadiuba was curious about its implementation in a new realm: the data collection itself. The LHC generates around 1 billion collisions a second, and physicists only have a few microseconds to decide which events are noteworthy and which can be discarded.
Ngadiuba wanted to integrate machine learning into this decision-making process but was at first hesitant. “I thought the LHC is too fast for machine learning,” she said. But this did not deter Ngadiuba and her colleagues. “The tools available from industry were not OK for us,” she said. “So let’s build our own.”
The result was a new tool called hls4ml, which allows physicists to program machine learning algorithms onto a type of chip called field-programmable gate arrays. Because FPGAs use parallel processing, they can analyze and reconstruct collision events much faster than traditional CPUs. The next step was finding a project in which they could deploy it.
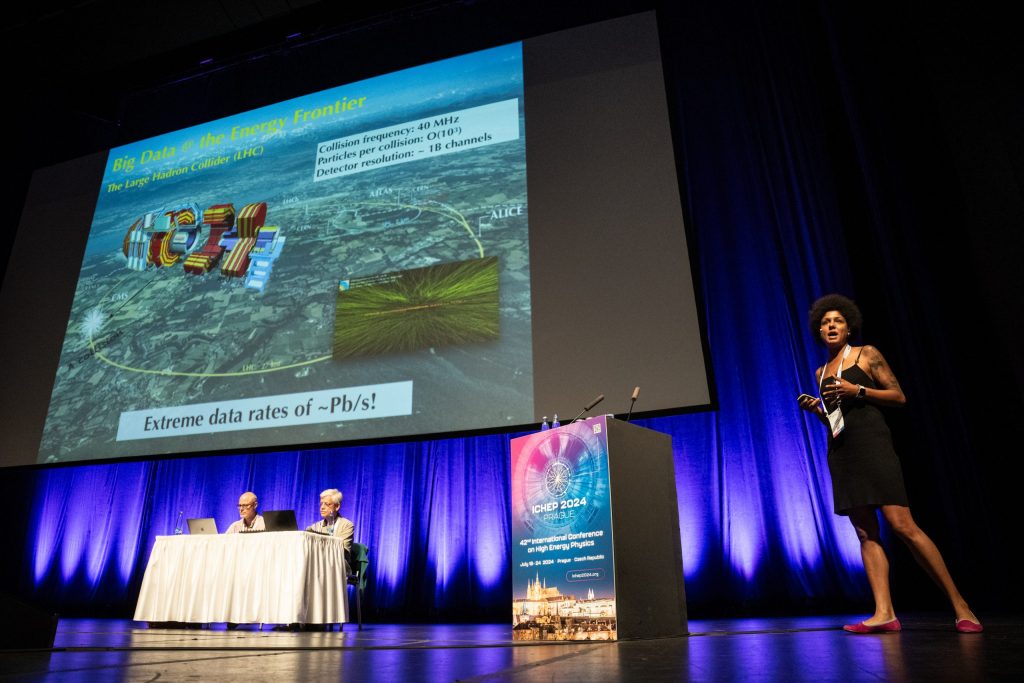
Jennifer Ngadiuba presents during the International Conference on High Energy Physics in Prague, where she was awarded the Early Career Scientist Prize in Particle Physics. Photo credit: Rene Volfik
“What’s the most interesting problem we want to tackle?” Ngadiuba said. “That’s why we decided to do anomaly detection.”
Most physics searches start with a theoretical model and then look for evidence to support that theory. In anomaly detection, physicists start by looking for odd things in the data.
“It’s a paradigm shift,” Ngadiuba said.
This past spring, Ngadiuba and her colleagues installed their anomaly detection tool in the CMS trigger system and have already collected their first data set.
“Many people write very interesting papers on what ATLAS and CMS could do with AI,” said Pierini, who has been working with Ngadiuba since she was a PhD student. “Jennifer doesn’t stop there. She pushes her idea forward, to real-life applications that change things for real, not just in principle.”
In addition to her work on CMS, Ngadiuba is currently partnering with physicists on the Deep Underground Neutrino Experiment to integrate the techniques developed by CMS into neutrino research.
Fermi National Accelerator Laboratory is supported by the Office of Science of the U.S. Department of Energy. The Office of Science is the single largest supporter of basic research in the physical sciences in the United States and is working to address some of the most pressing challenges of our time. For more information, please visit science.energy.gov.